If you're like many manufacturing leaders in the Midwest today, you're probably feeling the pressure to modernize while keeping costs under control. Let's face it—digital transformation isn't just a buzzword anymore, it's becoming essential for survival. In this guide, I'll break down how IoT digital twins can be game-changers for your business, whether you're running a small machine shop or managing multiple production facilities.
Is Big Data in Manufacturing Delivering Real Operational Value?

A Wisconsin-based auto parts manufacturer was facing a critical challenge: unexpected equipment failures were causing production delays, costing them over $2 million annually in downtime alone. After implementing sensors across their factory floor and analyzing the collected data, they identified subtle vibration patterns that predicted failures up to three weeks in advance. Within six months, they reduced unplanned downtime by 37% and saved an estimated $850,000—directly translating data insights into measurable operational value and bottom-line results.
Stories like this make big data in manufacturing sound like a silver bullet for achieving the core aspirations every manufacturer shares: operational efficiency, quality excellence, and competitive advantage. But for every success story, there are multiple manufacturing companies struggling to translate their data investments into meaningful innovation that delivers on these aspirations. Why?
The Promise vs. Reality of Big Data in Manufacturing
Big data in the manufacturing industry refers to the massive volumes of information generated throughout production processes, supply chains, and equipment operations. When leveraged effectively, this data drives operational efficiency through informed decision-making based on real-time insights rather than gut feelings or historical patterns.
The Three Core Aspirations of Modern Manufacturers
Before diving deeper, it's important to recognize that manufacturers consistently pursue three fundamental aspirations that drive their technology investments:
Operational Efficiency: Maximizing productivity while minimizing costs, downtime, and resource usage
Quality Excellence: Ensuring consistent product quality that meets or exceeds standards and customer expectations
Competitive Advantage: Differentiating from competitors through innovation, responsiveness, and superior market positioning
Big data, when powered by machine learning and AI, promises to deliver on all three aspirations. Based on this promise, industry publications and technology vendors paint a picture of data-driven manufacturing utopia: fully optimized operations, zero downtime, perfect quality control, and unprecedented innovation. The reality for many manufacturers, particularly in the Midwest's industrial heartland, is far more complex. D Large datasets without direction don't drive innovation or address these core aspirations—they create confusion, overwhelm teams, and drain resources.
The gap between promise and reality isn't about the technology itself, but rather how it's implemented. Successful data transformation in manufacturing depends on expert implementation that respects human expertise while leveraging technological capabilities. It's about enhancing, not replacing, the wisdom that experienced plant managers, line supervisors, and quality controllers bring to the manufacturing floor.
The Technology Ecosystem Powering Manufacturing Big Data
The manufacturing sector's big data revolution is built on a foundation of interconnected technologies:
Internet of Things (IoT) devices and sensors capture real-time data from every corner of the production floor. Modern manufacturing environments might contain thousands of these sensors monitoring temperature, pressure, vibration, energy consumption, movement, and countless other variables. These sensors generate unprecedented volumes of production data.
Machine learning algorithms analyze this flood of information to identify patterns and valuable insights human analysts would never discover. These systems excel at predicting outcomes based on complex interrelationships between hundreds of variables across the manufacturing process.
Artificial intelligence takes these insights further, making or recommending decisions based on analyzed data. In advanced implementations, AI can autonomously adjust machine parameters or production schedules to optimize efficiency.
Together, these technologies enable real-time decision-making at a scale previously impossible in manufacturing environments. Big data analytics process massive datasets instantly, allowing plant managers to respond to changing conditions as they emerge rather than after problems occur.
Where Big Data Delivers: Real Manufacturing Impact
When implemented strategically, big data transforms manufacturing operations across multiple dimensions:
Supply Chain Optimization
For manufacturers, supply chain disruptions represent one of the most significant operational risks. Big data contributes to supply chain management by enabling:
Real-time inventory tracking that reduces carrying costs while preventing stockouts
Supplier performance analysis that identifies reliability issues before they impact production
Predictive analytics that anticipate materials needs based on demand forecasting
Transportation optimization that reduces logistics costs and delivery times
A Michigan-based furniture manufacturer implemented data-driven inventory management that reduced their raw material holdings by 22% while improving on-time delivery performance from 87% to 96%. By applying big data analytics to their supply chain, they achieved both cost reduction and service improvement simultaneously.
Predictive Maintenance: The Cornerstone of Operational Excellence
Equipment failures represent a double threat to manufacturers: direct costs from repairs and indirect costs from production downtime. Traditional maintenance approaches either replace components prematurely (preventive) or wait until failure occurs (reactive). Neither is optimal.
Big data enables predictive maintenance by:
Identifying subtle shifts in equipment performance that precede failures
Scheduling maintenance during planned downtime periods
Extending equipment lifespan through optimized service intervals
Reducing spare parts inventory through more accurate forecasting
It's important to recognize that these predictive capabilities aren't inherent in the data itself. Rather, they emerge from sophisticated machine learning algorithms trained on historical failure patterns that can detect subtle deviations from normal operating conditions. Without these AI-powered analytical tools, even the most comprehensive sensor data would remain just numbers rather than actionable predictive insights.
According to McKinsey & Company's research, predictive maintenance typically reduces machine downtime by 30-50% and increases machine life by 20-40%. PwC's research indicates that predictive maintenance in manufacturing can reduce breakdown incidents by up to 70% and decrease maintenance costs by 25-30%. These efficiency gains directly translate to improved productivity and profitability—key metrics for operational excellence.
The manufacturing firms seeing the greatest ROI from predictive maintenance aren't just installing sensors and collecting data—they're combining this information with the contextual knowledge of experienced maintenance teams who understand each machine's quirks and history. Leading implementers leverage digital twin technologies that incorporate human expertise while transforming maintenance teams from reactive "fixers" to proactive "analyzers." The most effective systems establish clear boundaries between automated decisions and human oversight, ensuring the right balance of efficiency and judgment. This human-machine collaboration creates a powerful foundation for operational efficiency that neither could achieve alone.
Quality Control and Product Development: Achieving Quality Excellence
Manufacturing quality is fundamentally a data problem, and quality excellence remains a critical aspiration for manufacturers seeking to build customer loyalty and reduce costly defects and returns. Big data transforms quality control through:
Statistical process control with real-time adjustment capabilities
Automated visual inspection systems that detect defects human inspectors might miss
Root cause analysis that identifies systemic quality issues
Correlation of customer feedback with specific production variables
According to a recent study by LNS Research, manufacturers who have implemented quality 4.0 initiatives (which leverage big data analytics) saw a 64% reduction in the cost of poor quality and a 22% increase in new product introduction success rates. Research by Capgemini found that big data analytics in manufacturing quality processes improved detection of quality issues by 65% and reduced quality-related costs by up to 20%. These improvements directly enhance brand reputation, reduce warranty claims, and strengthen customer relationships.
In product development, big data enables precise market demands forecasting and product quality improvements by connecting customer usage patterns with design decisions. These actionable insights help manufacturers deliver products that truly meet customer needs—driving both quality excellence and competitive advantage through more targeted innovation.
Why Many Big Data Initiatives Fail in Manufacturing
Despite compelling success stories, many manufacturing big data initiatives fail to deliver promised results. Understanding these failure modes is essential to successful implementation:
Data Quality and Integration Challenges
The "garbage in, garbage out" principle applies powerfully in manufacturing analytics. Common data quality issues include:
Inconsistent data formats across different systems and sensors
Missing values from sensor malfunctions or connectivity issues
Uncalibrated sensors providing misleading readings
Timestamps that don't align across different data collection points
Integrating big data solutions with existing ERP (Enterprise Resource Planning) and MES (Manufacturing Execution Systems) presents additional challenges. Legacy systems often weren't designed to share data, creating silos that prevent holistic analysis. Many manufacturers also struggle with proprietary equipment protocols that don't adhere to modern connectivity standards, requiring custom integration solutions. This integration complexity is frequently underestimated, leading to implementation delays and budget overruns.
The Lost Knowledge Problem
Perhaps the most significant yet least discussed challenge is what we might call the "Lost Knowledge Problem"—when data implementation ignores human expertise acquired through years of hands-on experience.
Consider the maintenance technician who can diagnose equipment problems by subtle sounds or vibrations, the line supervisor who knows exactly how to adjust parameters based on material variations, or the quality inspector who can predict issues before they appear in measurements. When big data initiatives fail to capture and incorporate this tacit knowledge, they often deliver disappointing results despite technical sophistication.
The most successful implementations don't attempt to replace human expertise—they augment and scale it through thoughtfully designed systems that combine data insights with operational wisdom.
Bridging the Gap: From Data Collection to Operational Wisdom
Successful manufacturing data transformation follows a strategic implementation framework that begins with assessment before technology selection:
Assess organizational data maturity to understand current capabilities and gaps
Align data initiatives with specific business outcomes rather than implementing technology for its own sake
Develop a phased approach that delivers incremental value while building toward comprehensive capabilities
Progress through the DIKW journey—transforming Data into Information, Information into Knowledge, and Knowledge into Wisdom
The DIKW difference: transforming data into operational value
The Data-Information-Knowledge-Wisdom (DIKW) pyramid provides a powerful framework for understanding how manufacturers face different challenges at each stage of their data journey:

Data: the foundation challenge
At the data layer, manufacturers struggle with fragmented information trapped in siloed systems. Production data sits isolated from quality metrics, maintenance records remain disconnected from KPIs, and valuable knowledge walks out when experienced workers retire. Most companies collect whatever data is easiest to capture, missing critical insights hidden in "dark data" sources.
Information: the context challenge
Information challenges manifest when manufacturers can see data but can't make sense of it. Quality issues recur without clear root causes, maintenance remains reactive, and inefficiencies persist despite dashboards full of metrics. Traditional approaches create visualizations from readily available data without revealing meaningful connections between seemingly unrelated factors.
Knowledge: the prediction challenge
At the knowledge layer, manufacturers struggle to predict outcomes and understand why issues occur. Without modeling complex relationships between variables, they can't accurately forecast maintenance needs, product quality, or resource requirements. Many assume data alone can replace human judgment and institutional knowledge, missing the critical integration of expertise with analytics.
Wisdom: the decision challenge
Wisdom-level challenges occur when manufacturers need to make complex trade-offs at scale. Without systems that balance competing priorities like cost, quality, and speed, decision-makers become bottlenecks. The rush toward fully autonomous systems often removes valuable human oversight, creating rigidity where flexibility is needed.
Our approach addresses these challenges by creating data products that enhance human expertise rather than replacing it—preserving the wisdom that experienced operators and managers bring while leveraging technology to make it scalable and consistent. Expert guidance from manufacturing-specific domain experts is crucial, as generalist consultants often lack understanding of production environments' unique constraints.
Gaining Competitive Advantage Through Strategic Implementation
When properly implemented, big data systems transform manufacturing challenges into opportunities for innovation and market differentiation—directly supporting the core aspiration of competitive advantage. Research from IDC Manufacturing Insights shows that manufacturers leveraging big data analytics saw a 10-15% improvement in manufacturing efficiency, 15-20% increase in asset uptime, and 10-15% reduction in working capital requirements compared to industry peers.\According to the Manufacturing Institute, manufacturers implementing big data analytics reported significantly higher productivity growth compared to their non-data-driven counterparts. Supporting this market trend, recent McKinsey research found that AI and analytics could potentially create $1.2 trillion to $2 trillion in value annually in the manufacturing and supply chain sectors—a competitive edge that compounds over time for early adopters.
The competitive advantages manifest across multiple dimensions:
Speed-to-Market Advantage: Data-driven design and testing processes can reduce new product introduction times by 30-40%
Cost Position Advantage: Analytics-optimized production can lower overall production costs by 10-20%
Quality Reputation Advantage: Real-time quality monitoring with predictive capabilities can improve product consistency and performance
Responsiveness Advantage: Data-informed operations can adapt more quickly to changing market conditions and customer requirements
These competitive advantages aren't achieved through technology alone but through strategic partnerships that combine technical capabilities with manufacturing expertise. The sustained competitive advantage comes not just from the initial implementation but from ongoing collaboration that builds internal capabilities while continuously refining data systems based on operational feedback.
Realizing Big Data's Potential in Manufacturing: Fulfilling All Three Core Aspirations
Big data is indeed driving innovation in manufacturing—but only when implemented with strategic foresight and respect for operational expertise. The digital transformation journey requires both technological sophistication and deep understanding of manufacturing processes to deliver on manufacturers' core aspirations:
Operational Efficiency: As demonstrated by McKinsey research showing 30-50% downtime reduction and PwC findings of 25-30% maintenance cost decreases
Quality Excellence: Evidenced by LNS Research's documented 64% reduction in cost of poor quality and 22% increase in new product introduction success rates
Competitive Advantage: Supported by McKinsey's finding that AI and analytics could create $1.2-2 trillion in value annually in the manufacturing sector
As connectivity and data science continue to evolve, we'll see new and compelling use cases emerge. The manufacturers who successfully achieve their aspirations won't be those who simply adopt the latest technology but those who implement it strategically to enhance their unique operational strengths with expert guidance.
For manufacturing leaders, the key questions aren't about whether to embrace big data but when and how to implement it to deliver tangible operational value and bottom-line results. Success requires looking beyond technical capabilities to focus on outcomes that matter: improved quality, reduced costs, faster delivery, greater flexibility, and sustainable innovation.
The manufacturing operations that thrive in this data-rich environment will be those that use technology to amplify human expertise rather than replace it—creating systems where operators, engineers, and managers leverage data insights to make better decisions while contributing their irreplaceable contextual knowledge to the continuous improvement process. This balanced approach is what truly transforms data into operational wisdom and delivers on the promise of manufacturing innovation.
Is your manufacturing operation ready to transform data into operational value across all three core aspirations? Contact our data products experts for a no-obligation assessment of your organization's data maturity and opportunities.
About the Author:
Álan Gularte is a Senior Data Engineer at TXI in Chicago, where he transforms complex data into powerful solutions through innovative cloud engineering and machine learning approaches. He specializes in crafting strategic insights that help organizations like yours make data-driven decisions.
Published by Álan Gularte in Industry 4.0
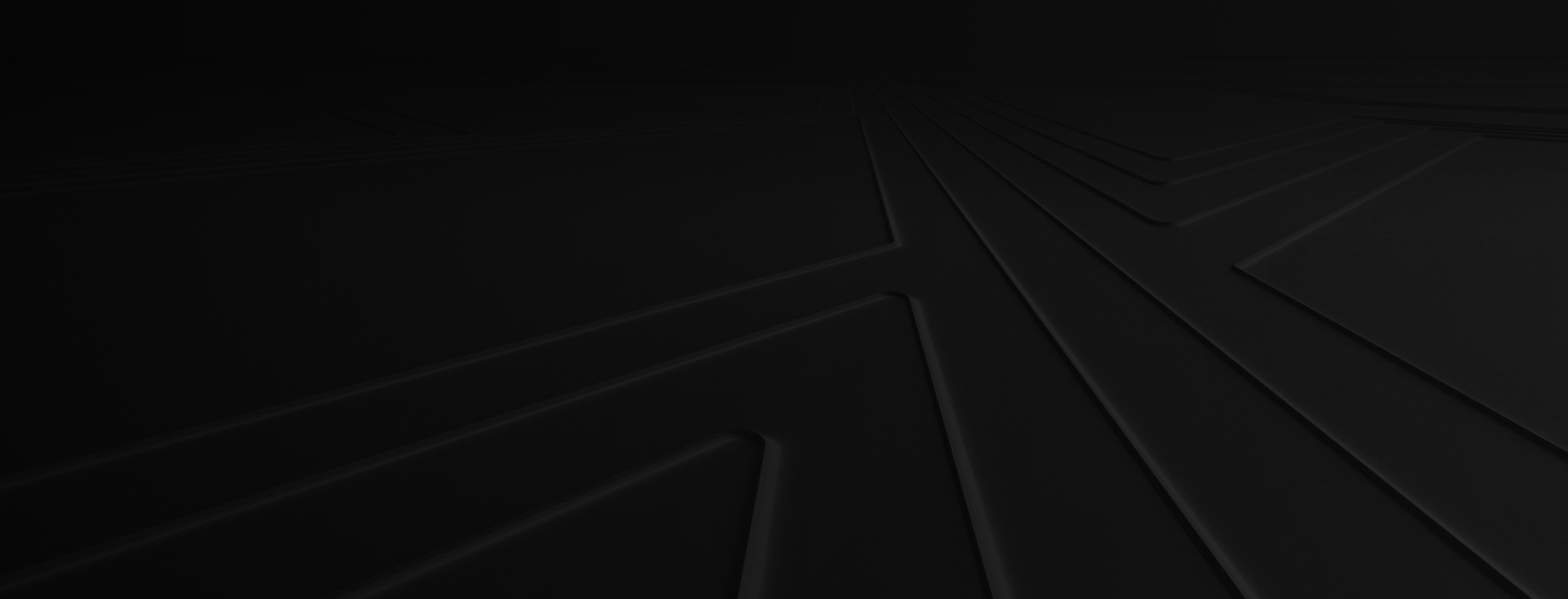
Let's shape your insights into experience-led data products together.