The future of the predictive maintenance
Modern Industrialist Podcast

Powered by RedCircle
The episode:
In episode 2 of The Modern Industrialist, Jason Hehman and co-host Turley delve into predictive maintenance options in the world of Industry 4.0. Tune in as they explore the foundations of predictive maintenance and discover how organizations use IoT sensor data to monitor system components and predict maintenance needs, ultimately saving money and reducing equipment downtime. We'll also cover the importance of diverse data sources and how computer vision can reveal hidden problems.
The podcast:
Presented by TXI, The Modern Industrialist Podcast is for technology-focused manufacturing and logistics leaders looking to gain a competitive edge with Industry 4.0 transformation. Join our host Jason Hehman as he brings together experts from companies blazing the path for the IIoT revolution. Topics range from advice to success stories, use cases, solutions, and more.
The expert:
Jason is the Vertical Lead for Industrial IoT and a Client Partner at TXI. With over 20 years of experience in the industry, he has certified brand strategist credentials and a proven track record of helping clients solve complex business problems through technology.
In Jason's current role, he works closely with clients in the industrial sector to help them leverage IoT to achieve growth and unlock new value. He applies expertise in product innovation, digital transformation, and smart manufacturing to guide them through every process step, from ideation to execution. He is passionate about understanding consumer behavior and using that insight to impact business strategy and create products that enhance user experience and satisfaction.
Podcast Host: Jason Hehman, Industry 4.0 Vertical Lead and Client Partner at TXI
Co-host: Patrick Turley, Head of Engineering at TXI
Book a meeting with Jason
Summary and themes explored in this episode:
Part 1: Understanding Predictive Maintenance
The Role of Technology in Predictive Maintenance
A recent Industry Week article sheds light on organizations' maintenance strategies.
- A survey by Dan Pena Associates revealed maintenance approaches:
63% favor planned maintenance schedules.
17% rely on reactive maintenance.
16% use predictive maintenance.
Defining Predictive Maintenance: Utilizing data from sensors, often through IoT, to monitor system components and predict maintenance needs.
It's more than guesswork; it's about leveraging technology and data for proactive maintenance.
Transforming Technician Deployment
Anecdote: A company in the industrial sector deploys technicians strategically across the US.
Predictive maintenance can optimize technician deployment, minimizing downtime.
Efficient deployment ensures technicians can respond swiftly to unexpected breakdowns.
Financial Impact of Maintenance Timing
Example: A railcar company in Chicago prioritizes minimizing maintenance downtime.
Predictive maintenance can pinpoint when maintenance is needed, maximizing revenue.
Real-time planning for workforce and locations enhances efficiency.
Part 2: Challenges and Considerations in Predictive Maintenance
Identifying Sensors and Establishing Baselines
The challenge: Choosing the right sensors and setting effective alarm thresholds.
Building a reliable baseline to detect anomalies is crucial for predictive maintenance success.
Incremental approach: Start with available data and expand as predictive models improve.
Enhancing Machines with Sensors
Data is key; sensors provide critical input for predictive models.
Mathematical strategies uncover hidden trends and patterns in data.
Continuous enhancement: The process is ongoing, not a one-time solution.
Balancing Strategy: Starting Small vs. Scaling
The dilemma: Start small for quick wins or gather enough data for reliable predictive models.
Importance of grassroots insights: Talk to experienced machine operators for unique insights.
Understanding operational quirks, like breakdowns on hot days, can inform sensor placement.
The Ongoing Journey of Predictive Maintenance
Predictive maintenance is not a one-time achievement but an evolving process.
Keep an eye on operational realities and adapt models to react effectively.
Predictive Maintenance Transcendence
The podcast explores the dynamic world of predictive maintenance.
Balancing the small-scale start with data-driven scaling is a recurring theme.
Predictive maintenance isn't about transcending but continually improving and adapting.
Part 3: Beyond the Factory Floor
Insights from the Frontlines
Often overlooked, frontline workers offer invaluable insights.
Incorporating operator experiences can enhance predictive models.
Worker input helps identify patterns and anomalies beyond sensors.
Diversifying Data Sources: Beyond Machines
Consider external data sources to supplement predictive models.
Environmental monitoring provides essential context outside machinery.
Factors like temperature and humidity impact machinery performance.
The Role of Computer Vision
Computer vision uncovers hidden issues beyond the naked eye.
Enhances predictive maintenance by detecting concealed problems.
Real-time insights from computer vision aid proactive solutions.
Human Input in Predictive Maintenance
Worker experiences provide valuable quantitative input.
Integrating operator feedback fine-tunes predictive models.
Even simple inputs, like emotional feedback, offer insights.
Digital Twins vs. Digital Shadows
Digital twins replicate equipment or processes digitally.
Digital shadows provide one-way data flow, offering performance snapshots.
True digital twinning involves two-way data interaction and real-time adjustments.
Custom Software and Sensor Integration
Both building and buying are pivotal in predictive maintenance.
Custom software integrates with off-the-shelf sensors.
A holistic approach combines purchased components and tailored solutions.
Striking the Build vs. Buy Balance
The build vs. buy decision often leans toward both.
Mixing off-the-shelf components with custom solutions offers flexibility.
Agile approaches with partner considerations are key to success.
Embracing the Predictive Maintenance Journey
The podcast delves into the vital aspects of predictive maintenance.
Discusses challenges like data complexity and decision-making.
Encourages audience engagement and sharing experiences in this evolving field.
Published by Jason Hehman , Patrick Turley in podcasts
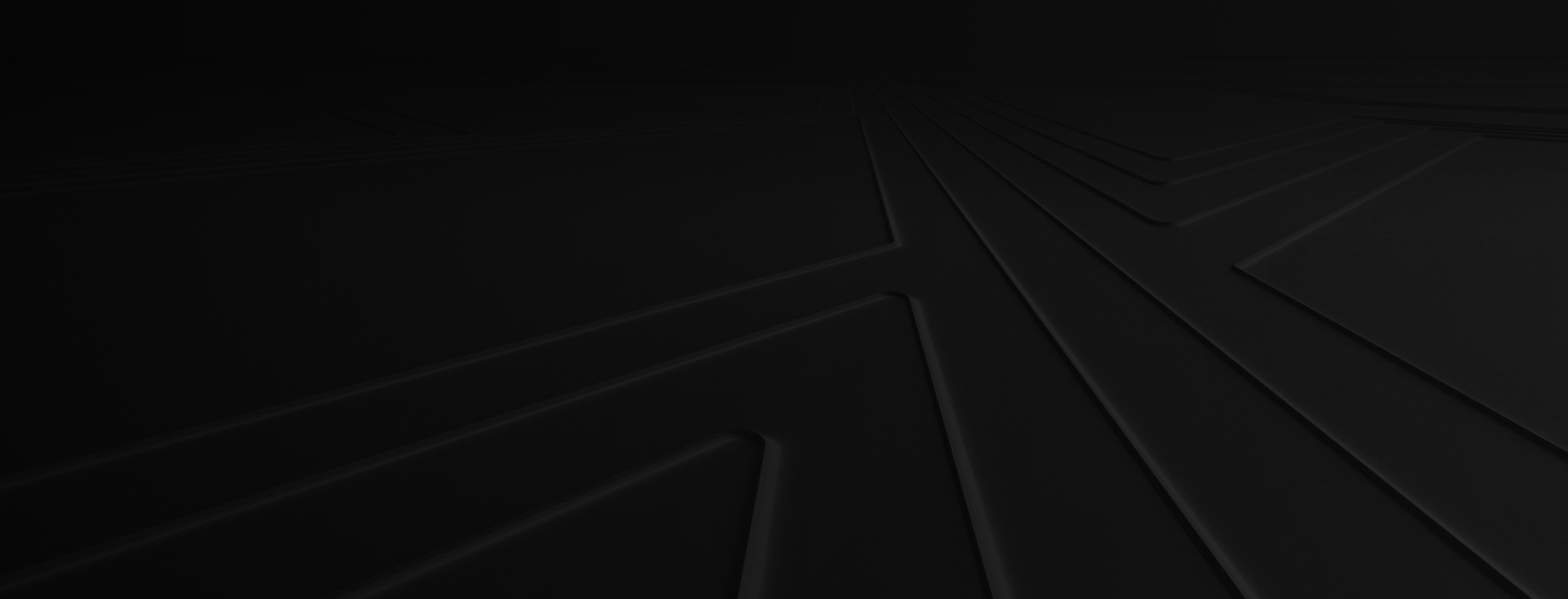
Let's shape your insights into experience-led data products together.