More than 80% of AI projects fail. See how mid-market leaders are investing in AI—and what’s holding others back.
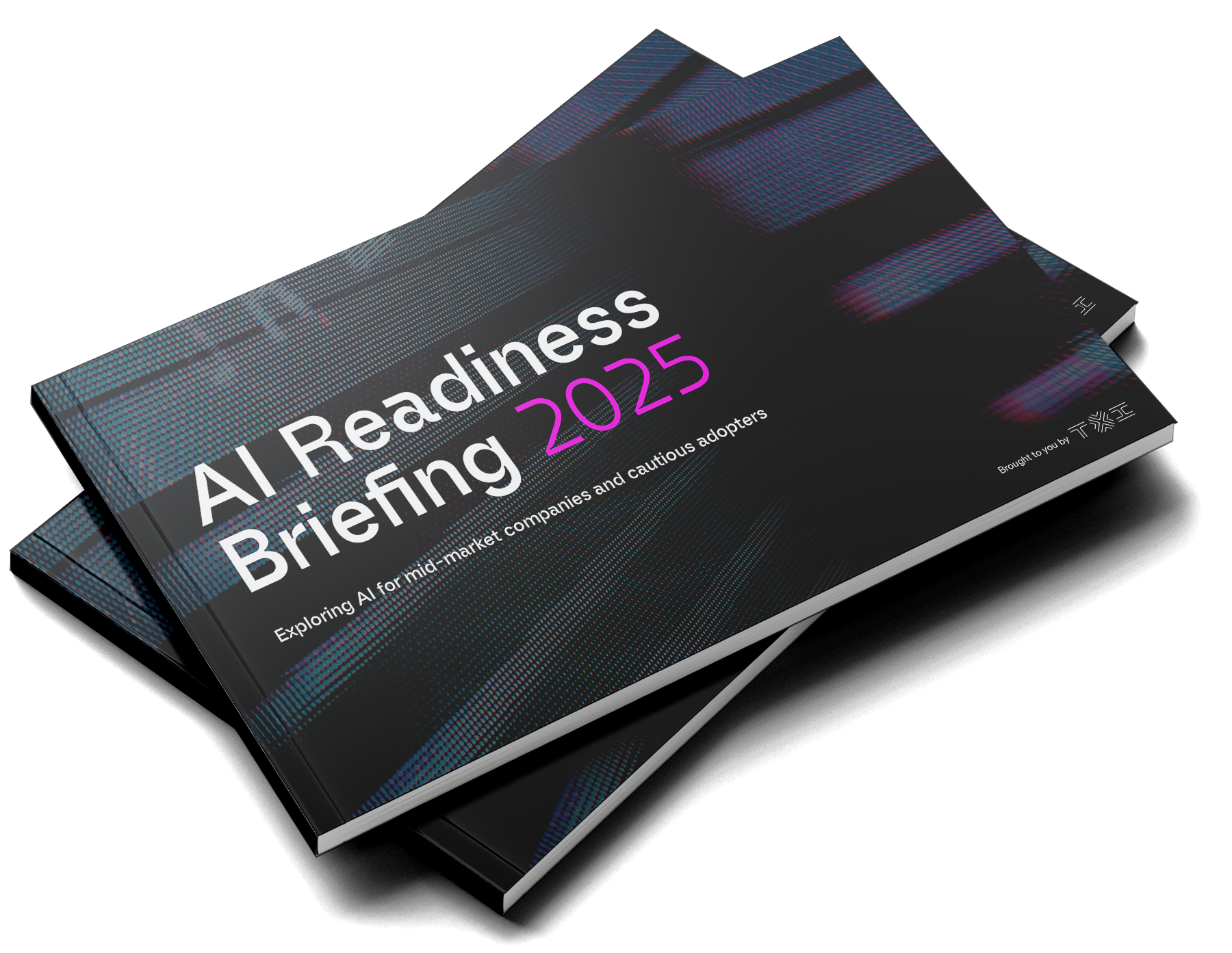
More than 80% of AI projects fail. See how mid-market leaders are investing in AI—and what’s holding others back.
Mid-market orgs looking to explore AI responsibly
Leaders tasked with transformation, innovation, or operational efficiency
Companies unsure about the cost of implementing AI and how to start smart
You'll understand:
This isn’t another AI hype cycle piece. It’s grounded, strategic, and built for cautious adopters ready to take a first step.
TXI is a digital product consultancy helping mid-market orgs design, build, and scale impactful AI solutions—with a strong focus on cost efficiency, ethical implementation, and long-term value.
Being AI ready means your organization has the necessary infrastructure, data maturity, and operational alignment to effectively implement artificial intelligence and machine learning technologies to drive real-world outcomes.
Generative AI adds value when it’s applied with a clear purpose — not just because it’s trending. For mid-market organizations, it can accelerate internal content creation (think manuals, documentation, or training guides), improve customer support workflows, and even support faster prototyping. But the real value comes when it’s built on clean data, integrated into existing processes, and guided by human oversight. Without that, it’s just noise.
Many AI initiatives fail due to the “Lost Knowledge Problem”—when AI projects prioritize data over human expertise, leading to poor execution and loss of operational insight. In the report, we present an implementation framework that addresses this issue.
AI use cases include predictive maintenance, process optimization, quality control, and workflow automation—especially when integrated with domain-specific knowledge and context-aware datasets.
AI models thrive on rich, relevant datasets. Capturing “dark data”—hidden operational insights or undocumented expertise—can significantly improve model performance and practical applicability. In the report, learn how to harness this data effectively.
GenAI (generative AI) creates new content such as text, images, or code based on learned patterns, whereas traditional machine learning focuses on prediction and classification based on existing data.
Yes—especially in regards to where it could create leverage. Staying competitive doesn’t mean jumping on every new tool but it is absolutely worth knowing where AI fits (and doesn’t), and having the foundation to act when the time is right. The organizations that win won’t be the fastest adopters—they’ll be the ones who align AI with real problems, data they trust, and teams who know how to act on the output.
The key is designing decision support tools that complement human oversight—enhancing, rather than replacing, experiential wisdom with intelligent recommendations.
Successful AI projects require cross-functional teams, operational alignment, trustworthy data, and systems that scale—not just tech sophistication but also cultural and workflow integration.
AI literacy ensures that your teams understand the capabilities and limitations of AI, enabling them to collaborate more effectively with technologists and make informed decisions about automation.
By building modular, interpretable, and human-centered AI systems that evolve with your needs—prioritizing explainability, adaptability, and collaboration between digital innovators and industry veterans.